This is part one of a series on statistical methods for analysing time-to-event, or "survival" data.
Key takeaways
This post explains some fundamental concepts in infectious disease research, including how we measure disease spread and severity:
- How we track how many people acquire an infection (incidence) and how many people have the infection at one time (prevalence)
- What the "$R$ number" tells us about disease spread
- Different methods to assess the impact of disease when we don't have perfect data
- Challenges in measuring the true burden of infectious diseases
Epidemiological measures
Epidemiology is the study and analysis of patterns of diseases and determinants of health in a population. In the context of infectious diseases, epidemiologists and statisticians employ several standard measures of disease burden to assess the impact of a disease, and to guide the appropriate public health response. These measures include:
- the incidence of infection, defined as the rate of new infections occurring over a specific period of time, e.g. new cases per 1,000 people per year;
- the prevalence of infection, defined as the proportion of the population that have the disease at a specific point in time, regardless of when they became infected;
- for diseases with adverse outcomes, the mortality associated with infection, defined as the incidence of death from the disease.
The $R$ number
A key measure of the spread of a disease, related to the incidence of infection, is the basic reproduction number, denoted $R_0$. The $R_0$ value measures the expected number of cases directly generated by one case in a population where all individuals are susceptible to infection.
For instance, if $R_0 = 3$ for a particular disease then one infected individual will, on average, infect three others. When $R_0 > 1$ an infection will spread in a population, whereas when $R_0 > 1$ the infection will eventually die out.
Importantly, not every individual within a population will be equally susceptible to infection. Immunity to a pathogen might be acquired through vaccination or recovery from previous infection, both of which can provide a level of protection against future infections. Through widespread vaccination, immunity can be conferred to a large portion of a population, reducing susceptibility and thereby decreasing $R_0$.
Estimating the burden of infectious disease
To understand how quickly a virus is spreading through a population, and the corresponding burden of disease, timely and robust estimates are required. The incidence of new cases, prevalence of undiagnosed infection, and extent of disease severity can all inform policy makers seeking to implement effective and appropriate public health measures.
Incidence and prevalence
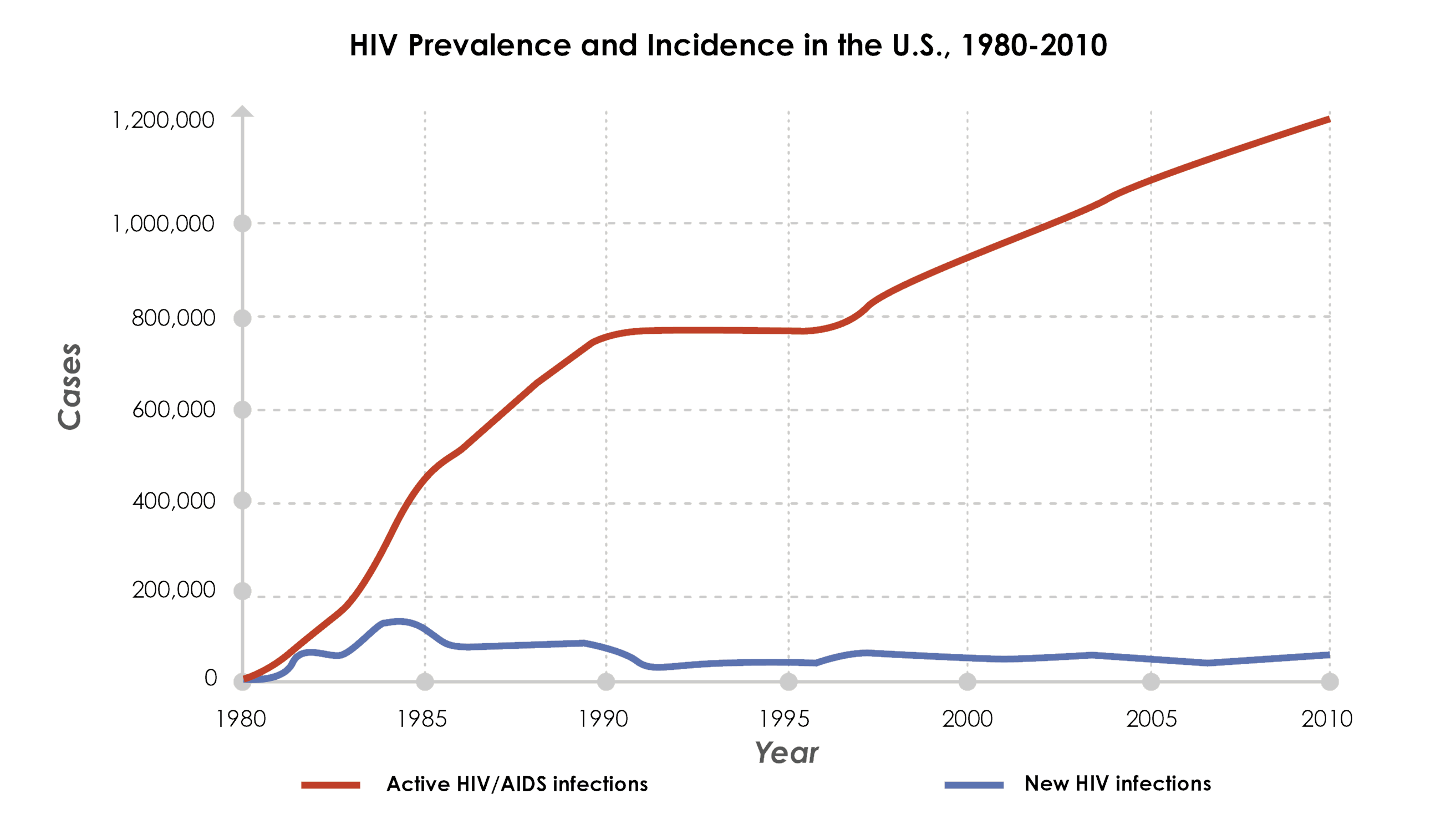
Figure 1: An example of the prevalence and incidence of HIV in the US over time. Incidence measures new cases over time, while prevalence measures total active cases at a specific point. Figure from: Steward K.
Incidence of infection and the prevalence of an infectious disease in a population are generally unobserved, with observable information restricted to test outcomes among subsets of a population. Testing processes may themselves be influenced by unobserved parameters, such as an individual's propensity to test, and the time from infection to symptom presentation (if symptoms present at all).
To reconstruct the incidence and prevalence of infection from the available testing data, statistical and mathematical modelling techniques which adjust for sampling biases are employed. These techniques combine information from a range of sources to make valid statistical inference about unobserved incidence and prevalence.
Severity
Two common metrics used to understand the severity of infectious diseases and the factors associated with adverse outcomes are the infection fatality risk (IFR) and case fatality risk (CFR):
- Infection Fatality Risk (IFR): The percentage of all infected people who die from the disease (including those whose infections were never detected)
- Case Fatality Rate (CFR): The percentage of confirmed cases who die from the disease
Since infections resulting in death are more likely to be recorded, and mild infections (particularly asymptomatic infections) less likely to be recorded, the CFR will almost always be higher than the IFR. Statistical modelling can be used to relate these measures of severity to underlying characteristics collected through case-surveillance data.
Effectiveness of interventions
Monitoring outcomes among a target group after the introduction of a public health intervention allows for the real-world effectiveness of the intervention to be assessed, and changes over time to be detected. For example, effectiveness estimates might consider reductions in the transmission or acquisition of an infection among a treated group compared to an untreated group. Making these estimates requires reliable testing data, recognition of potential biases, and methodologies which can account for these biases.
Challenges in estimating infectious disease burden
The majority of the data available for infectious disease monitoring relies on observational data, where a population is followed without actively intervening on an exposure in a randomised way. As a result, estimates of infectious disease burden are often considerably complicated by selection bias, which arises when the selected population observed is different from the general population of interest.
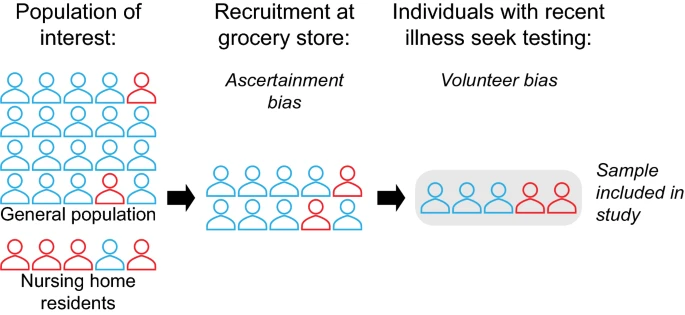
Figure 2: Schematic showing recruitment-based biases in a hypothetical serosurvey. Figure from: Accorsi et al.
Common causes of selection bias in observational data include: testing policies (which may vary nationally and sub-nationally), individual health-seeking behaviour, test availability, clinician decision-making, and detection rates of different tests. Inaccuracies in the available case-surveillance data may also arise: at data input, due to incomplete/inaccurate reporting or misdiagnosis; and at data collection, due to insufficient surveillance infrastructure and data processing methods.
Unless these potential sources of bias are well-understood and accounted for they can affect the validity of estimates. For example, the IFR measure of severity requires complete knowledge of both the number of infections and number of deaths. Particularly in the early stages of a pandemic, when testing is either unavailable or sub-optimal, these quantities may be impossible to measure. The CFR is more straightforward to assess, since confirmed infections are more likely to be recorded, but this measure is highly sensitive to testing practices. This was particularly the case during the initial wave of the COVID-19 pandemic, when biases in case-surveillance led to wide variability in national estimates of the case-fatality rate, from 0.1% to over 25%.
Even when knowledge of these multi-faceted biases is available, statistical methodologies must be carefully chosen to properly control for the biases.
List of key terms
- Epidemiology
- The study of how diseases spread and affect populations
- Incidence
- The rate of new cases in a population over a specific period (e.g. 50 new cases per 1,000 people per year)
- Prevalence
- The total proportion of a population affected by a condition at a specific point in time
- $R_0\ $ (Basic reproduction number)
- The average number of people one infected person will transmit the disease to in a completely susceptible population
- IFR (Infection Fatality Risk)
- The percentage of infected individuals (including those with undetected infections) who die from the disease
- CFR (Case Fatality Rate)
- The percentage of officially confirmed cases that result in death
- Selection bias
- When the data collected doesn't accurately represent the entire population of interest
Coming next
In the next post I'll provide an introduction to survival analysis, time-to-event data, and how we handle censored observations in epidemiological research.
References
- Accorsi EK, Qiu X, Rumpler E, et al. How to detect and reduce potential sources of biases in studies of SARS-CoV-2 and COVID-19. Eur J Epidemiol. 2021;36(2):179-96.
- De Angelis D, Presanis AM, Birrell PJ, et al. Four key challenges in infectious disease modelling using data from multiple sources. Epidemics. 2015;10:83-7.
- Lipsitch M, Cohen T, Cooper B, et al. Transmission dynamics and control of severe acute respiratory syndrome. Science. 2003;300(5627):1966-70.
- Perez-Guzman PN, Knock E, Imai N, et al. Epidemiological drivers of transmissibility and severity of SARS-CoV-2 in England. Nat Commun. 2023;14(1):4279.
- Steward K. Incidence vs Prevalence. Immunology & Microbiology from Technology Networks. Technology Networks; 2020.
- Westreich D. Berkson's bias, selection bias, and missing data. Epidemiology. 2012;23(1):159-64.
- World Health Organization. Estimating mortality from COVID-19 (Scientific Brief). World Health Organization; 2020.
For more details about key concepts in epidemiology and infectious disease research, see:
- Epidemiology: Study design and data analysis by Mark Woodward